资深k8s工程师,深入解读OpenAI宕机事故真相,以及应对策略
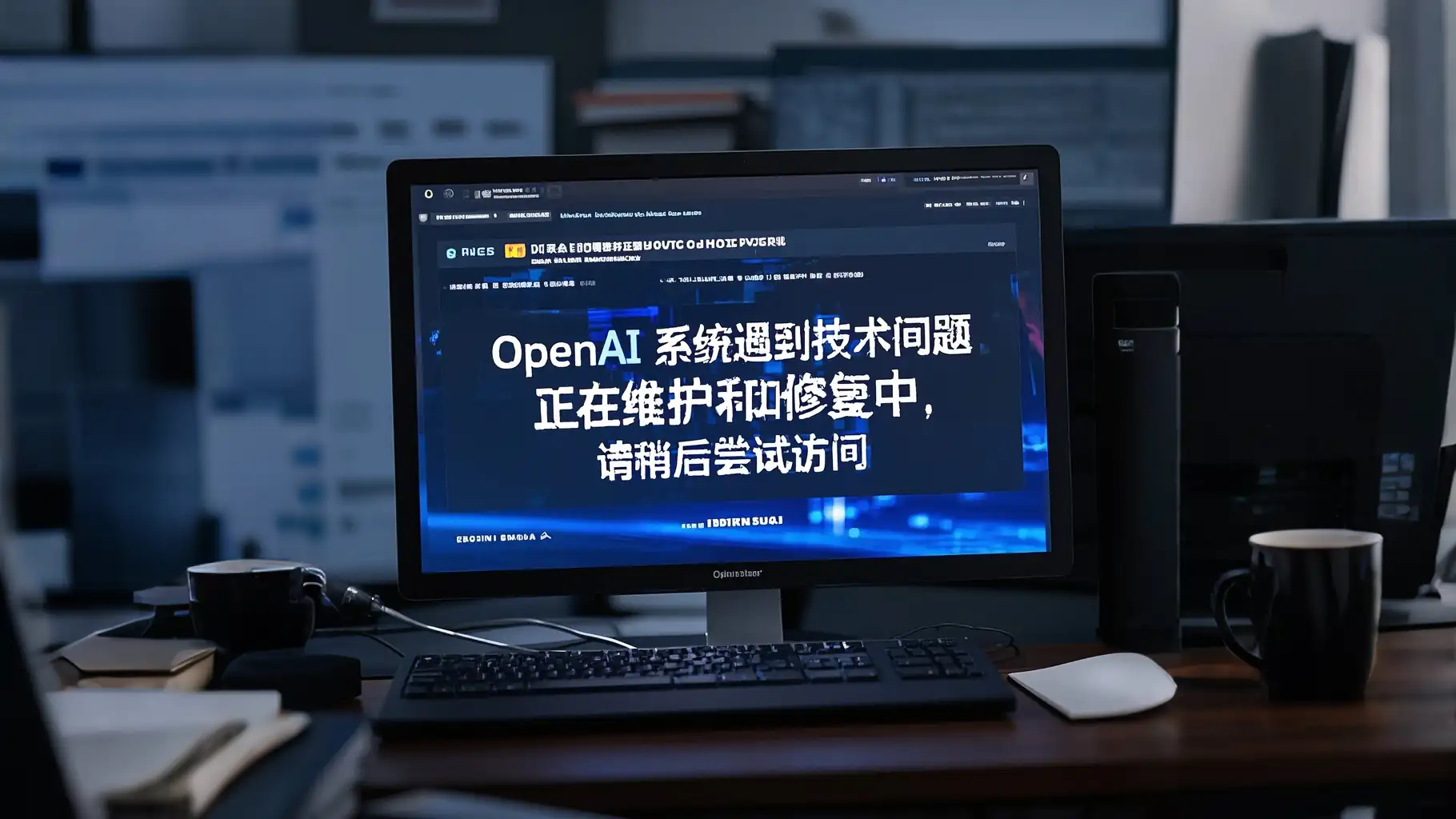
太平洋时间2024年12月11日,OpenAI发生了严重故障,主要原因是其Kubernetes集群控制平面崩溃。对于外行人来说,可能只是看看热闹,但作为内行,我从技术角度来分析一下这个故障。
我在看完事故报告时候,产生3个疑问?
- Telemetry服务是做什么的?
- 大量的API请求(expensive requests )是什么请求?为什么这个程序会导致大量的资源敏感API请求?
- 为什么会影响业务系统?
1 Telemetry服务是做什么的
报告中提到:
At 3:12 PM PST, we deployed a new telemetry service to collect detailed Kubernetes control plane metrics.
Telemetry services have a very wide footprint, so this new service’s configuration unintentionally caused every node in each cluster to execute resource-intensive Kubernetes API operations whose cost scaled with the size of the cluster. With thousands of nodes performing these operations simultaneously, the Kubernetes API servers became overwhelmed, taking down the Kubernetes control plane in most of our large clusters.
从这段话中,我们可以提取到几个关键信息:
- Telemetry服务的目的是收集Kubernetes控制平面的监控数据(metrics)。
- 这个服务的配置不小心让每个节点都执行了“expensive requests”。
- 随着几千个节点同时发起这些请求,Kubernetes的API服务器被压垮,导致控制平面崩溃。
根据上面的信息我推断,它所定义的控制平面除了apiserver、controller-maneger、scheduler,还包含kubelet。而这个Telemetry会去收集各个节点上kubelet的监控信息。
关于Telemetry服务的部署方式,可能有两种可能性:
- Push模式:每个节点上部署一个agent,收集节点的监控数据,然后上报给中心服务。
- Pull模式:一个中心服务定期拉取每个节点上的kubelet监控数据。
根据openAI的kubernetes集群规模达到7500节点文章里提到*”We try to avoid having any DaemonSets interact with the API Server. “*,所以我推测使用的是第二种Pull模式。
2 大量的API请求是什么请求?
在Kubernetes中,所谓的“expensive requests”通常是指list
请求,因为它们会消耗大量的内存。如果数据不在watch cache
中,还会加重etcd的压力。
但从部署架构来看,每个节点上不太可能部署一个pod来发起这些list
请求。
那应该是什么请求?
我的答案是认证和授权两个请求。以openAI对安全的要求,kubelet会关闭匿名请求(--anonymous-auth=false
),且会开启认证和授权(--authentication-token-webhook
和--authorization-mode=Webhook
)。
具体来说,kubelet会在收到请求访问其提供的接口(如/metrics/*
、/stats/*
、/logs/*
)时,向API Server发送TokenReview和SubjectAccessReview请求来进行认证和授权。
一般apiserver会开启多种认证模式(不同场景需要不同的认证方式),这样认证必须走固定的流程(RequestHeader 、Basic auth、x509、static token、service account token、bootstrap token、OIDC、webhook、Anonymous)。如果开启oidc和webhook还要调用第三方系统,链路会更长。
kubelet开启授权后是通常也会设置--authorization-webhook-cache-authorized-ttl
和--authorization-webhook-cache-unauthorized-ttl
,用于缓存认证结果。但是第一请求的话,kubelet中不会有缓存。
在这种情况下,当Telemetry服务向大量节点抓取监控数据时,就会同时触发kubelet向API Server发起大量的认证和授权请求。
3 为什么会影响业务系统?
在Kubernetes中,即使apiserver宕机,控制平面的其他组件(controller-manager、scheduler)和数据平面的组件(kubelet、kube-proxy、pod)仍然能继续运行,容器也不会重启。只要业务不依赖Kubernetes的服务(比如依赖Kubernetes的service域名),业务本身不会受到影响。甚至ingress controller,市面上的ingress controller都有很好的鲁棒性。
但是,OpenAI的业务使用了Kubernetes的服务发现机制,即它依赖集群内部的DNS来解析服务的域名。
报告中提到的:
The impact was specific to clusters exceeding a certain size, and our DNS cache on each node delayed visible failures long enough for the rollout to continue.
…
DNS caching mitigated the impact temporarily by providing stale but functional DNS records. However, as cached records expired over the following 20 minutes, services began failing due to their reliance on real-time DNS resolution.
根据这个可以推断出,OpenAI的集群可能采用了Nodelocal DNS和CoreDNS的机制,其中Nodelocal DNS充当本地缓存,缓存时间为20分钟。
具体来说:
如果请求的域名在Nodelocal dns缓存中,直接返回缓存结果。
如果不在Nodelocal dns缓存中的域名,如果不在缓存中,它会向CoreDNS发起请求,CoreDNS会查询对应的service或endpoint,返回ClusterIP或所有endpoint的ip。
当apiserver挂掉,它只会影响informer中数据更新(无法感知service和endpoint和endpointslice变化),实际上CoreDNS仍然能继续提供DNS解析。
总结一下
对于域名对应的是headless的service,coredns解析是所有endpoint对应的ip。否则,service解析成ClusterIP。
当apiserver宕机时,Coredns解析服务依然正常运行,只是这个解析数据可能是过时的记录。
3.1 报告中对于dns描述存疑
报告中提到:
DNS caching mitigated the impact temporarily by providing stale but functional DNS records. However, as cached records expired over the following 20 minutes, services began failing due to their reliance on real-time DNS resolution. This timing was critical because it delayed the visibility of the issue, allowing the rollout to continue before the full scope of the problem was understood. Once the DNS caches were empty, the load on the DNS servers was multiplied, adding further load to the control plane and further complicating immediate mitigation.
但我对这段话有些疑问:如果服务依赖实时解析,为什么要启用DNS缓存呢?
而且,只有在以下两种情况下,DNS问题才会导致业务中断:
- 现有服务的Rollout或Scale:这个时候service对应的endpoint、endpointslice发生变化。如果是headless service,那么coredns解析出来的ip是过时的。如果是clusterIP,那么解析出来的ip不会发生变化。但是因为kube-proxy没有同步endpoint,导致clusterIP流量转发错误。
- 新的业务发布,它使用新的service。那么coredns无法解析这个域名,其他服务在尝试访问它时才会发现问题。
因此,我认为合理的解释是DNS缓存只是故障的其中一个因素,真正的根本原因可能是架构设计上存在缺陷,dns只是一个替罪羊。
4 如何救火
当apiserver宕机时,集群的组件和客户端通常会采用指数退避算法来重新连接apiserver,这会加剧API服务器的压力。
这个时候想移除Telemetry服务的pod也无能为力,因为无法连接上apiserver。即使连上apiserver,执行了删除操作,也要controller-mamanger和kubelet连上apiserver,且能够成功执行相应的操作。
OpenAI的应急响应包括:
- 缩小集群规模:减少请求的压力。
- 从网络层屏蔽客户端访问:防止不必要的请求冲击apiserver。
- 扩容apiserver:增强apiserver的处理能力。
思路是尽可能降低apiserver的负载,让apiserver从故障中恢复。然后扩容apiserver,让不断重试的客户端和集群组件能够连上apiserver,同时保证连上之后,不会再次打挂apiserver。
5 如何避免类似故障?改进措施
主要思路:应该避免将底层平台与业务系统过于耦合,避免依赖kubernetes的服务发现系机制,不要使用configmap作为微服务的配置中心。
以下建议基于kubernetes v1.30版本
5.1 客户端
使用新版本的client-go:因为社区在不断的优化Refector,采用新的版本能有效减少压力。
限制客户端对apiserver的并发量:为集群组件设置合适的–kube-api-burst 和–kube-api-qps,为所有基于client-go开发的客户端设置合适的qps和burst。
5.2 API Server
分组apiserver,分为集群组件使用和管理维护使用(包括发布系统)。这样在集群出现问题时候,管理操作仍能进行。
启用API Priority and Fairness(APF): API Priority and Fairness 根据优先级和公平性完成请求的排队和调度,这个默认启用。
配置合理的
PriorityLevelConfiguration
和FlowSchema
,保证在系统压力大时候,重要的请求不会被不重要的请求压垮,确保重要的请求能够得到apiserver的响应。设置合理的最大请求并发数:在启用APF功能时,apiserver的最大并发数是由
--max-requests-inflight
(默认400) 和--max-mutating-requests-inflight
(默认200)的总和决定的。使用单独的etcd来保存event事件: 比如–etcd-servers-overrides=/events#http://172.18.0.2:14379
设置合理的watch cache大小:watch cache(
--watch-cache-sizes
和--default-watch-cache-size
)是用于apiserver缓存etcd中数据,太小容易导致list请求从etcd中读取,太大会消耗内存Caution在1.25版本之后,–watch-cache-sizes设置非0值代表开启这类资源的watch cache且不能设置大小(使用默认值),0代表关闭watch cache https://github.com/kubernetes/kubernetes/pull/109201
启用goaway-chance:在客户端通过负载均衡器来访问apiserver时候,让多个apiserver的长连接均衡。 如果客户端http/2长连接不断开,则会导致多个节点apiserver的长连接数和负载不均衡。开启这个参数后,apiserver会随机给HTTP/2 客户端发送 GOAWAY 帧,客户端收到后会断开连接,然后客户端重新与apiserver建立连接,这样负载均衡算法就能随机选择一个apiserver,最终多个apiserver的长连接会均衡。
5.3 社区中正在优化的东西
在1.31和1.32版本中增加了WatchList,让list请求使用stream进行传输,极大的提高效率,并减少内存消耗,它的目标替换reflector中list watch机制。
在1.31版本中Resilient watchcache initialization 解决watch cache没有在apisever启动的时候初始化和需要重新初始化的场景。
我有多年资深的kubernetes经验,提供kubernetes问题的解答,故障的排查、源码解读等咨询服务。点击这里联系我